1、小别墅三层装修效果图中的尚层一站式品质整装有何独特之处
小别墅三层装修效果图中的尚层一站式品质整装的独特之处:
1. 全屋设计与施工一站式服务:
尚层提供从设计到施工的一站式服务,无需业主再找多家公司,大大简化装修流程。
2. 专业设计师团队:
拥有资深的设计师团队,为业主提供个性化、符合需求的设计方案。
3. 精选优质材料:
精选知名品牌环保材料,保障室内健康和舒适性。
4. 标准化施工流程:
严格执行标准化施工流程,确保工程质量和施工效率。
5. 严格质量把控:
采用多重质检程序,严格把控施工质量,保证最终装修效果。
6. 专业售后服务:
提供完善的售后服务,及时解决业主提出的问题,保障入住后的安心。
具体到小别墅三层装修效果图中的展示:
一层: 宽敞的客厅,落地窗带来充足采光,提升空间感。
开放式厨房,与餐厅无缝衔接,打造流畅动线。
卧室温馨舒适,搭配落地窗,享受自然美景。
二层: 主卧套房,私密性好,设有宽敞衣帽间和豪华卫浴。
儿童房,充满童趣,满足孩子的想象力。
书房,安静舒适,打造阅读和工作空间。
三层: 阳光房,采光极佳,可用于休闲或健身。
露台,视野开阔,享受户外惬意时光。
多功能室,可根据业主需求定制为娱乐室、影音室等。
尚层一站式品质整装以专业的设计、优质的材料、严苛的施工和完善的服务,为业主打造高品质、省心省力的装修体验。
2、三层小别墅装修大概需要多少钱
三层小别墅的装修费用取决于多种因素,包括建筑面积、装修档次、所选材料、人工成本和当地市场情况。以下是一些影响装修费用因素的概览:
1. 建筑面积:别墅面积越大,装修费用越高。
2. 装修档次:装修档次分为经济型、标准型、中高档和高档,档次越高,装修费用越高。
3. 所选材料:材料的选择直接影响装修成本。例如,使用实木地板比使用复合地板成本更高。
4. 人工成本:不同地区的劳动力成本差异很大。人工成本高的地区,装修费用也会更高。
5. 当地市场情况:
装修费用还受当地市场供需关系的影响。在旺季或供需不平衡的情况下,装修费用会更高。
根据以上因素,三层小别墅的装修费用范围可以从几十万元到上百万元不等。更具体的费用可以咨询当地的装修公司或设计师,他们可以根据你的具体情况提供准确的报价。
以下是三层小别墅装修不同档次的估算费用:
经济型:600900元/平方米
标准型:元/平方米
中高档:元/平方米
高档:1800元/平方米以上
注意:上述费用仅供参考,实际费用可能因不同情况而有所差异。建议在装修前咨询多家装修公司,比较报价,并选择性价比最高的方案。
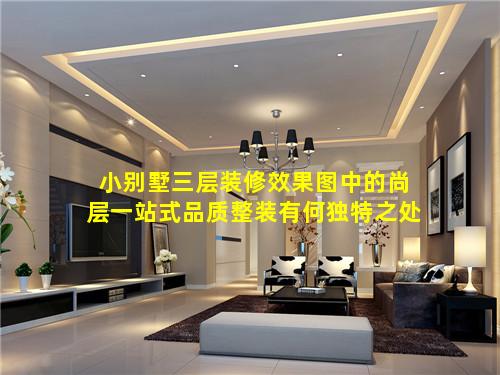
3、小别墅装修效果图全图
Data Science and Machine Learning Methods for Data Analytics and Interpretation in Neuroimaging
Abstract:
Neuroimaging techniques have revolutionized our understanding of the brain and its functions. However, the analysis and interpretation of neuroimaging data presents significant challenges due to its high dimensionality, noise, and complex structure. Data science and machine learning (ML) methods provide powerful tools for addressing these challenges and extracting meaningful insights from neuroimaging data. This paper reviews the application of data science and ML methods for data analytics and interpretation in neuroimaging. We discuss various data preprocessing and feature extraction techniques, supervised and unsupervised learning algorithms, and their use in disease diagnosis, prognosis, and treatment planning. We highlight recent advances and emerging trends in the field, and provide insights into best practices and future directions for research and development.
Introduction:
Neuroimaging techniques, such as functional magnetic resonance imaging (fMRI), electroencephalography (EEG), and magnetoencephalography (MEG), allow us to noninvasively measure brain activity and structure. These techniques have led to significant advancements in our understanding of brain function and dysfunction, providing insights into neurological and psychiatric disorders, cognitive processes, and brain development.
However, the analysis and interpretation of neuroimaging data is challenging due to its high dimensionality, noise, and complex structure. Traditional statistical methods often fall short in handling these complexities, leading to limited interpretability and reduced accuracy in data analysis.
Data Science and Machine Learning Methods for Neuroimaging:
Data science and ML methods offer powerful tools for addressing the challenges in neuroimaging data analysis and interpretation. These methods enable researchers to:
Preprocess and extract meaningful features from raw neuroimaging data.
Identify patterns and relationships in data using supervised and unsupervised learning algorithms.
Develop predictive models for disease diagnosis, prognosis, and treatment planning.
Data Preprocessing and Feature Extraction:
Data preprocessing is essential to prepare neuroimaging data for analysis. Techniques such as image registration, noise removal, and artifact correction ensure that data is consistent and comparable across subjects. Feature extraction aims to transform raw data into informative features that capture relevant information for analysis. Common feature extraction methods include:
Regionofinterest (ROI) analysis: Extracting data from predefined brain regions.
Voxelbased morphometry (VBM): Analyzing differences in brain structure based on voxel intensities.
Diffusion tensor imaging (DTI): Measuring the diffusion of water molecules to assess white matter integrity.
Electroencephalography (EEG) and magnetoencephalography (MEG): Extracting timeseries data of brain electrical or magnetic activity.
Supervised and Unsupervised Learning Algorithms:
Supervised learning algorithms require labeled data (e.g., disease diagnosis) to train models that can classify or predict outcomes. Common supervised learning algorithms used in neuroimaging include:
Support vector machines (SVMs): Classifying data based on hyperplanes that maximize the margin between different classes.
Random forests: Combining multiple decision trees to improve classification accuracy.
Neural networks: Complex models inspired by the human brain that can learn hierarchical representations of data.
Unsupervised learning algorithms do not require labeled data and are used to identify patterns and relationships in unlabeled data. Common unsupervised learning algorithms used in neuroimaging include:
Principal component analysis (PCA): Reducing the dimensionality of data by identifying principal components that capture most of the variance.
Cluster analysis: Grouping data into clusters based on similarity measures.
Independent component analysis (ICA): Identifying independent components that represent distinct sources of activity in the brain.
Applications in Disease Diagnosis, Prognosis, and Treatment Planning:
Data science and ML methods have been successfully applied in various areas of neuroimaging research, including:
Disease diagnosis: Classifying individuals with neurological or psychiatric disorders based on neuroimaging data.
Prognosis: Predicting the progression of a disease and its response to treatment.
Treatment planning: Optimizing treatment strategies for individual patients based on their neuroimaging profiles.
Recent Advances and Emerging Trends:
Recent advances in data science and ML have led to the development of new methods and techniques for neuroimaging data analysis. These include:
Deep learning: Neural network models with multiple layers that can learn complex hierarchical representations of data.
Generative models: Models that can generate new synthetic data that is similar to real neuroimaging data.
Transfer learning: Transferring knowledge from models trained on large datasets to smaller datasets in neuroimaging.
Emerging trends include the application of data science and ML methods to:
Big data in neuroimaging: Analyzing largescale neuroimaging datasets to identify rare and subtle patterns.
Personalized medicine: Developing precision medicine approaches by tailoring treatments to individual patients based on their neuroimaging profiles.
Neuroimaging biomarkers: Identifying neuroimaging features that can serve as diagnostic or prognostic biomarkers for various disorders.
Best Practices and Future Directions:
To ensure the effective use of data science and ML methods in neuroimaging, researchers should adhere to best practices, such as:
Careful data collection and preprocessing: Ensuring data quality and consistency.
Principled feature extraction: Selecting features that are relevant to the research question and interpretable.
Appropriate model selection and validation: Choosing algorithms that are suitable for the data and task, and evaluating model performance rigorously.
Interpretation and validation of results: Interpreting ML models carefully and validating findings using independent data.
Future directions for research and development include:
Developing more interpretable ML models: Making ML models more transparent and understandable to improve confidence in their predictions.
Integrating multiple neuroimaging modalities: Combining data from different neuroimaging techniques to provide a more comprehensive understanding of brain function.
Exploring new applications: Expanding the use of data science and ML methods to address unmet needs in neuroimaging research and clinical practice.
Conclusion:
Data science and ML methods play a crucial role in the analysis and interpretation of neuroimaging data. These methods have transformed our ability to extract meaningful insights from complex and highdimensional neuroimaging data. By leveraging data science and ML, researchers can advance our understanding of the brain, improve diagnostics and prognostics, and develop personalized medical treatments for neurological and psychiatric disorders. Continued research and development in this field will further empower us to harness the vast potential of neuroimaging data for improving human health and wellbeing.
4、小别墅二层装修效果图
[二层小别墅室内装修效果图]